4 changed files with 295 additions and 0 deletions
@ -0,0 +1,55 @@ |
|||||||
|
#!/usr/bin/env python |
||||||
|
# -*- coding: utf-8 -*- |
||||||
|
""" |
||||||
|
Copyright (c) 2022 PX4 Development Team |
||||||
|
Redistribution and use in source and binary forms, with or without |
||||||
|
modification, are permitted provided that the following conditions |
||||||
|
are met: |
||||||
|
|
||||||
|
1. Redistributions of source code must retain the above copyright |
||||||
|
notice, this list of conditions and the following disclaimer. |
||||||
|
2. Redistributions in binary form must reproduce the above copyright |
||||||
|
notice, this list of conditions and the following disclaimer in |
||||||
|
the documentation and/or other materials provided with the |
||||||
|
distribution. |
||||||
|
3. Neither the name PX4 nor the names of its contributors may be |
||||||
|
used to endorse or promote products derived from this software |
||||||
|
without specific prior written permission. |
||||||
|
|
||||||
|
THIS SOFTWARE IS PROVIDED BY THE COPYRIGHT HOLDERS AND CONTRIBUTORS |
||||||
|
"AS IS" AND ANY EXPRESS OR IMPLIED WARRANTIES, INCLUDING, BUT NOT |
||||||
|
LIMITED TO, THE IMPLIED WARRANTIES OF MERCHANTABILITY AND FITNESS |
||||||
|
FOR A PARTICULAR PURPOSE ARE DISCLAIMED. IN NO EVENT SHALL THE |
||||||
|
COPYRIGHT OWNER OR CONTRIBUTORS BE LIABLE FOR ANY DIRECT, INDIRECT, |
||||||
|
INCIDENTAL, SPECIAL, EXEMPLARY, OR CONSEQUENTIAL DAMAGES (INCLUDING, |
||||||
|
BUT NOT LIMITED TO, PROCUREMENT OF SUBSTITUTE GOODS OR SERVICES; LOSS |
||||||
|
OF USE, DATA, OR PROFITS; OR BUSINESS INTERRUPTION) HOWEVER CAUSED |
||||||
|
AND ON ANY THEORY OF LIABILITY, WHETHER IN CONTRACT, STRICT |
||||||
|
LIABILITY, OR TORT (INCLUDING NEGLIGENCE OR OTHERWISE) ARISING IN |
||||||
|
ANY WAY OUT OF THE USE OF THIS SOFTWARE, EVEN IF ADVISED OF THE |
||||||
|
POSSIBILITY OF SUCH DAMAGE. |
||||||
|
|
||||||
|
File: frag_fusion_symbolic.py |
||||||
|
Author: Mathieu Bresciani <mathieu@auterion.com> |
||||||
|
License: BSD 3-Clause |
||||||
|
Description: |
||||||
|
""" |
||||||
|
|
||||||
|
from sympy import * |
||||||
|
|
||||||
|
V = Symbol("V", real=True) |
||||||
|
rho = Symbol("rho", real=True) |
||||||
|
rho_n = Symbol("rho_n", real=True) |
||||||
|
Mcoef = Symbol("Mcoef", real=True) |
||||||
|
Bcoef = Symbol("Bcoef", real=True) |
||||||
|
a = Symbol("a", real=True) |
||||||
|
|
||||||
|
f1 = 0.5 * rho / Bcoef * V**2 + rho_n * Mcoef * V - a |
||||||
|
|
||||||
|
print("If Bcoef > 0 and Mcoef > 0") |
||||||
|
print("V =") |
||||||
|
res_V = solve(f1, V) |
||||||
|
res_V = res_V[0] |
||||||
|
pprint(res_V) |
||||||
|
print("a_pred =") |
||||||
|
pprint(solve(f1, a)) |
@ -0,0 +1,202 @@ |
|||||||
|
#!/usr/bin/env python |
||||||
|
# -*- coding: utf-8 -*- |
||||||
|
""" |
||||||
|
Copyright (c) 2022 PX4 Development Team |
||||||
|
Redistribution and use in source and binary forms, with or without |
||||||
|
modification, are permitted provided that the following conditions |
||||||
|
are met: |
||||||
|
|
||||||
|
1. Redistributions of source code must retain the above copyright |
||||||
|
notice, this list of conditions and the following disclaimer. |
||||||
|
2. Redistributions in binary form must reproduce the above copyright |
||||||
|
notice, this list of conditions and the following disclaimer in |
||||||
|
the documentation and/or other materials provided with the |
||||||
|
distribution. |
||||||
|
3. Neither the name PX4 nor the names of its contributors may be |
||||||
|
used to endorse or promote products derived from this software |
||||||
|
without specific prior written permission. |
||||||
|
|
||||||
|
THIS SOFTWARE IS PROVIDED BY THE COPYRIGHT HOLDERS AND CONTRIBUTORS |
||||||
|
"AS IS" AND ANY EXPRESS OR IMPLIED WARRANTIES, INCLUDING, BUT NOT |
||||||
|
LIMITED TO, THE IMPLIED WARRANTIES OF MERCHANTABILITY AND FITNESS |
||||||
|
FOR A PARTICULAR PURPOSE ARE DISCLAIMED. IN NO EVENT SHALL THE |
||||||
|
COPYRIGHT OWNER OR CONTRIBUTORS BE LIABLE FOR ANY DIRECT, INDIRECT, |
||||||
|
INCIDENTAL, SPECIAL, EXEMPLARY, OR CONSEQUENTIAL DAMAGES (INCLUDING, |
||||||
|
BUT NOT LIMITED TO, PROCUREMENT OF SUBSTITUTE GOODS OR SERVICES; LOSS |
||||||
|
OF USE, DATA, OR PROFITS; OR BUSINESS INTERRUPTION) HOWEVER CAUSED |
||||||
|
AND ON ANY THEORY OF LIABILITY, WHETHER IN CONTRACT, STRICT |
||||||
|
LIABILITY, OR TORT (INCLUDING NEGLIGENCE OR OTHERWISE) ARISING IN |
||||||
|
ANY WAY OUT OF THE USE OF THIS SOFTWARE, EVEN IF ADVISED OF THE |
||||||
|
POSSIBILITY OF SUCH DAMAGE. |
||||||
|
|
||||||
|
File: drag_replay.py |
||||||
|
Author: Mathieu Bresciani <mathieu@auterion.com> |
||||||
|
License: BSD 3-Clause |
||||||
|
Description: |
||||||
|
Find the best ballistic and momentum drag coefficients for wind estimation |
||||||
|
using EKF2 replay data. |
||||||
|
NOTE: this script currently assumes no wind. |
||||||
|
""" |
||||||
|
|
||||||
|
import matplotlib.pylab as plt |
||||||
|
from pyulog import ULog |
||||||
|
from pyulog.px4 import PX4ULog |
||||||
|
import numpy as np |
||||||
|
import quaternion |
||||||
|
from scipy import optimize |
||||||
|
|
||||||
|
def getAllData(logfile): |
||||||
|
log = ULog(logfile) |
||||||
|
|
||||||
|
v_local = np.matrix([getData(log, 'vehicle_local_position', 'vx'), |
||||||
|
getData(log, 'vehicle_local_position', 'vy'), |
||||||
|
getData(log, 'vehicle_local_position', 'vz')]) |
||||||
|
|
||||||
|
t_v_local = ms2s(getData(log, 'vehicle_local_position', 'timestamp')) |
||||||
|
|
||||||
|
accel = np.matrix([getData(log, 'sensor_combined', 'accelerometer_m_s2[0]'), |
||||||
|
getData(log, 'sensor_combined', 'accelerometer_m_s2[1]'), |
||||||
|
getData(log, 'sensor_combined', 'accelerometer_m_s2[2]')]) |
||||||
|
t_accel = ms2s(getData(log, 'sensor_combined', 'timestamp')) |
||||||
|
|
||||||
|
q = np.matrix([getData(log, 'vehicle_attitude', 'q[0]'), |
||||||
|
getData(log, 'vehicle_attitude', 'q[1]'), |
||||||
|
getData(log, 'vehicle_attitude', 'q[2]'), |
||||||
|
getData(log, 'vehicle_attitude', 'q[3]')]) |
||||||
|
t_q = ms2s(getData(log, 'vehicle_attitude', 'timestamp')) |
||||||
|
|
||||||
|
dist_bottom = getData(log, 'vehicle_local_position', 'dist_bottom') |
||||||
|
t_dist_bottom = ms2s(getData(log, 'vehicle_local_position', 'timestamp')) |
||||||
|
|
||||||
|
(t_aligned, v_body_aligned, accel_aligned) = alignData(t_v_local, v_local, t_accel, accel, t_q, q, t_dist_bottom, dist_bottom) |
||||||
|
|
||||||
|
t_aligned -= t_aligned[0] |
||||||
|
|
||||||
|
return (t_aligned, v_body_aligned, accel_aligned) |
||||||
|
|
||||||
|
def alignData(t_v, v_local, t_accel, accel, t_q, q, t_dist_bottom, dist_bottom): |
||||||
|
len_accel = len(t_accel) |
||||||
|
len_q = len(t_q) |
||||||
|
len_db = len(t_dist_bottom) |
||||||
|
i_a = 0 |
||||||
|
i_q = 0 |
||||||
|
i_db = 0 |
||||||
|
v_body_aligned = np.empty((3,0)) |
||||||
|
accel_aligned = np.empty((3,0)) |
||||||
|
t_aligned = [] |
||||||
|
|
||||||
|
for i_v in range(len(t_v)): |
||||||
|
t = t_v[i_v] |
||||||
|
accel_sum = np.zeros((3,1)) |
||||||
|
accel_count = 0 |
||||||
|
while t_accel[i_a] < t and i_a < len_accel-1: |
||||||
|
accel_sum += accel[:, i_a] # Integrate accel samples between 2 velocity samples |
||||||
|
accel_count += 1 |
||||||
|
i_a += 1 |
||||||
|
while t_q[i_q] < t and i_q < len_q-1: |
||||||
|
i_q += 1 |
||||||
|
while t_dist_bottom[i_db] < t and i_db < len_db-1: |
||||||
|
i_db += 1 |
||||||
|
|
||||||
|
# Only use in air data |
||||||
|
if dist_bottom[i_db] < 1.0 or accel_count == 0: |
||||||
|
continue |
||||||
|
|
||||||
|
qk = np.quaternion(q[0, i_q],q[1, i_q],q[2, i_q],q[3, i_q]) |
||||||
|
q_vl = np.quaternion(0, v_local[0, i_v], v_local[1, i_v], v_local[2, i_v]) |
||||||
|
q_vb = qk.conjugate() * q_vl * qk # Get velocity in body frame |
||||||
|
vb = quaternion.as_float_array(q_vb)[1:4] |
||||||
|
|
||||||
|
v_body_aligned = np.append(v_body_aligned, [[vb[0]], [vb[1]], [vb[2]]], axis=1) |
||||||
|
accel_aligned = np.append(accel_aligned, accel_sum / accel_count, axis=1) |
||||||
|
t_aligned.append(t) |
||||||
|
|
||||||
|
return (t_aligned, v_body_aligned, np.asarray(accel_aligned)) |
||||||
|
|
||||||
|
def getData(log, topic_name, variable_name, instance=0): |
||||||
|
variable_data = np.array([]) |
||||||
|
for elem in log.data_list: |
||||||
|
if elem.name == topic_name: |
||||||
|
if instance == elem.multi_id: |
||||||
|
variable_data = elem.data[variable_name] |
||||||
|
break |
||||||
|
|
||||||
|
return variable_data |
||||||
|
|
||||||
|
def ms2s(time_ms): |
||||||
|
return time_ms * 1e-6 |
||||||
|
|
||||||
|
def run(logfile): |
||||||
|
(t, v_body, a_body) = getAllData(logfile) |
||||||
|
|
||||||
|
rho = 1.15 # air densitiy |
||||||
|
rho15 = 1.225 # air density at 15 degC |
||||||
|
|
||||||
|
# x[0]: momentum drag, scales with v |
||||||
|
# x[1]: inverse of ballistic coefficient (X body axis), scales with v^2 |
||||||
|
# x[2]: inverse of ballistic coefficient (Y body axis), scales with v^2 |
||||||
|
predict_acc_x = lambda x: -v_body[0] * x[0] - 0.5 * rho * v_body[0]**2 * np.sign(v_body[0]) * x[1] |
||||||
|
predict_acc_y = lambda x: -v_body[1] * x[0] - 0.5 * rho * v_body[1]**2 * np.sign(v_body[1]) * x[2] |
||||||
|
|
||||||
|
J = lambda x: np.sum(np.power(abs(a_body[0]-predict_acc_x(x)), 2.0) + np.power(abs(a_body[1]-predict_acc_y(x)), 2.0)) # cost function |
||||||
|
|
||||||
|
x0 = [0.15, 1/100, 1/100] # initial conditions |
||||||
|
res = optimize.minimize(J, x0, method='nelder-mead', bounds=[(0,1),(0,10),(0,10)], options={'disp': True}) |
||||||
|
|
||||||
|
# Convert results to parameters |
||||||
|
innov_var = J(res.x) / (len(v_body[0]) + len(v_body[1])) |
||||||
|
mcoef = res.x[0] / np.sqrt(rho / rho15) |
||||||
|
|
||||||
|
bcoef_x = 0.0 |
||||||
|
bcoef_y = 0.0 |
||||||
|
|
||||||
|
if res.x[1] > 1/200: |
||||||
|
bcoef_x = 1/res.x[1] |
||||||
|
|
||||||
|
if res.x[2] > 1/200: |
||||||
|
bcoef_y = 1/res.x[2] |
||||||
|
|
||||||
|
print(f"param set EKF2_BCOEF_X {bcoef_x:.1f}") |
||||||
|
print(f"param set EKF2_BCOEF_Y {bcoef_y:.1f}") |
||||||
|
print(f"param set EKF2_MCOEF {mcoef:.2f}") |
||||||
|
print(f"/!\EXPERIMENTAL param set EKF2_DRAG_NOISE {innov_var:.2f}") |
||||||
|
|
||||||
|
# Plot data |
||||||
|
plt.figure(1) |
||||||
|
plt.suptitle(logfile.split('/')[-1]) |
||||||
|
ax1 = plt.subplot(2, 1, 1) |
||||||
|
ax1.plot(t, v_body[0]) |
||||||
|
ax1.plot(t, v_body[1]) |
||||||
|
ax1.set_xlabel("time (s)") |
||||||
|
ax1.set_ylabel("velocity (m/s)") |
||||||
|
ax1.legend(["forward", "right"]) |
||||||
|
|
||||||
|
ax2 = plt.subplot(2, 1, 2, sharex=ax1) |
||||||
|
ax2.set_title(f"BCoef_x = {bcoef_x:.1f}, BCoef_y = {bcoef_y:.1f}, MCoef = {mcoef:.4f}", loc="right") |
||||||
|
ax2.plot(t, a_body[0]) |
||||||
|
ax2.plot(t, a_body[1]) |
||||||
|
ax2.plot(t, predict_acc_x(res.x)) |
||||||
|
ax2.plot(t, predict_acc_y(res.x)) |
||||||
|
ax2.set_xlabel("time (s)") |
||||||
|
ax2.set_ylabel("acceleration (m/s^2)") |
||||||
|
ax2.legend(["meas_forward", "meas_right", "predicted_forward", "predicted_right"]) |
||||||
|
plt.show() |
||||||
|
|
||||||
|
if __name__ == '__main__': |
||||||
|
import os |
||||||
|
import argparse |
||||||
|
|
||||||
|
# Get the path of this script (without file name) |
||||||
|
script_path = os.path.split(os.path.realpath(__file__))[0] |
||||||
|
|
||||||
|
# Parse arguments |
||||||
|
parser = argparse.ArgumentParser( |
||||||
|
description='Estimate mag biases from ULog file') |
||||||
|
|
||||||
|
# Provide parameter file path and name |
||||||
|
parser.add_argument('logfile', help='Full ulog file path, name and extension', type=str) |
||||||
|
args = parser.parse_args() |
||||||
|
|
||||||
|
logfile = os.path.abspath(args.logfile) # Convert to absolute path |
||||||
|
|
||||||
|
run(logfile) |
@ -0,0 +1,32 @@ |
|||||||
|
# PX4 Drag fusion parameter tuning algorithm |
||||||
|
In PX4, drag fusion can be enabled in order to estimate the wind when flying a multirotor, assuming that the body vertical acceleration is produced by the rotors and that the lateral forces are produced by drag. |
||||||
|
The model assumes a combination of: |
||||||
|
1. momentum drag: created by the rotors changing the direction of the incoming air, linear to the relative airspeed. Parameter `EKF2_MCOEF` |
||||||
|
2. bluff body drag: created by the wetted area of the aircraft, quadratic to the relative airspeed. Parameters `EKF2_BCOEF_X` and `EKF2_BCOEF_Y` |
||||||
|
|
||||||
|
The python script was created to automate the tuning of the aforementioned parameters using flight test data. |
||||||
|
|
||||||
|
## How to use this script |
||||||
|
First, a flight log with enough information is required in order to accurately estimate the parameters. |
||||||
|
The best way to do this is to fly the drone in altitude mode, accelerate to a moderate-high speed and let the drone slow-down by its own drag. |
||||||
|
Repeat the same maneuver in all directions and several times to obtain a good dataset. |
||||||
|
|
||||||
|
/!\ NOTE: the current state of this script assumes no wind. Some modifications are required to estimate both the wind and the parameters at the same time. |
||||||
|
|
||||||
|
Then, install the required python packages: |
||||||
|
``` |
||||||
|
pip install -r requirements.txt |
||||||
|
``` |
||||||
|
and run the script and give it the log file as an argument: |
||||||
|
``` |
||||||
|
python drag_replay.py <logfilename.ulg> |
||||||
|
``` |
||||||
|
|
||||||
|
The estimated parameters are displayed in the console and the fit quality is shown in a plot: |
||||||
|
``` |
||||||
|
param set EKF2_BCOEF_X 0.0 |
||||||
|
param set EKF2_BCOEF_Y 62.1 |
||||||
|
param set EKF2_MCOEF 0.16 |
||||||
|
/!\EXPERIMENTAL param set EKF2_DRAG_NOISE 0.31 |
||||||
|
``` |
||||||
|
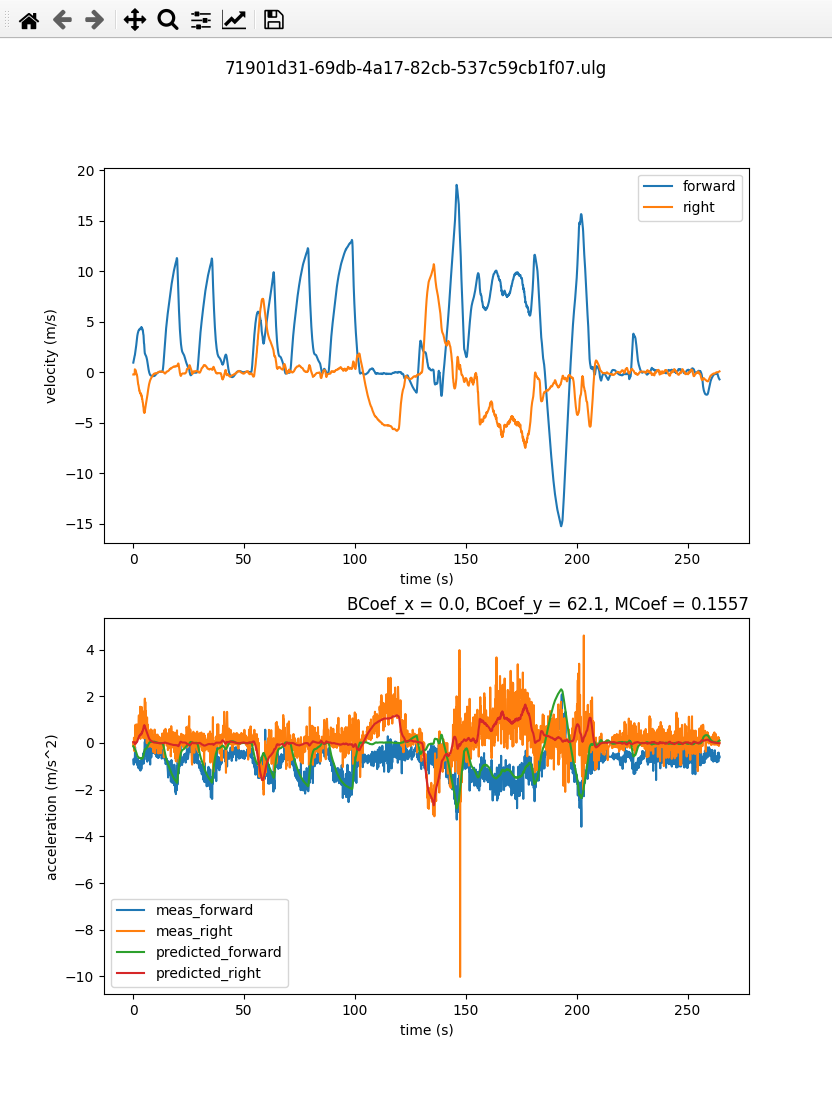 |
Loading…
Reference in new issue